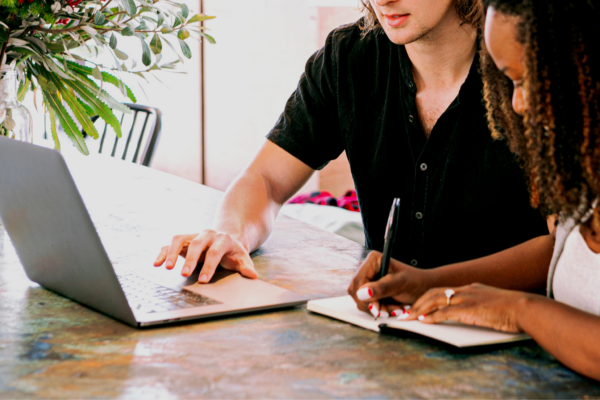
Prioritize Your A/B Tests Like The Experts: An Interview With Experimentation Leader Natalie Thomas
There is no “one size fits all” A/B test prioritization method. So, we asked experimentation leader Natalie Thomas to share more about how product marketing and ecommerce teams can pick the right method for them and how The Good prioritizes using the ADVIS’R Prioritization Framework™️.
Product marketing and ecommerce teams need A/B testing frameworks and prioritization models. That’s a fact.
Following a structured process for prioritizing optimizations has tons of benefits including:
- Making better decisions
- Assuring the success of your experimentation program
- Overcoming internal politics
But, what is not a fact is that there is a “best way to prioritize a/b tests.”
Whether it’s PIE or ICE A/B testing framework, there are plenty of experts out there who will argue that their prioritization model is best. And while the Google results might tell you otherwise, there is no single best prioritization model.
Here is the long and the short of it. The best prioritization framework is not necessarily simple, binary, or numerical. The best prioritization model is the one that works for your team. That’s it. If it sticks, it fits.
After reading one too many “The Best Way to Prioritize Your A/B Tests” articles, we sat down with our one of our in-house user experience and digital journey optimization experts, Natalie Thomas, to get her thoughts on the topic.
Keep reading to learn how to find the right prioritization model for you, what to think about when prioritizing, what prioritization model our team uses, and why the framework works well for us.
I understand there is no “one size fits all” for A/B testing framework and prioritization, but how can teams assess if a method is right for them?
The right prioritization model is going to depend on the factors of your organization:
- If your team is low trust, a system based on firm calculations that mitigate in-fighting might be best for you.
- If leadership is strongly concerned with the ROI of your program and you’re still in the proof-of-concept phase for your team, then money saved or won may be more heavily weighted than other aspects.
- If you are a resource-light team working to gain proof-of-concept, a prioritization method that heavily weighs the speed of results might give you the traction you need to make a case for greater investment.
- If your product struggles with churn, you may want to prioritize user needs over other factors in order to build a stable of happy customers and word-of-mouth traction.
The list goes on! As we say, there is no single approach is the best for everyone.
Let’s take The Good as a case study. How did you decide on a prioritization method that worked best for your clients?
When we started doing optimization, we were early practitioners. There weren’t flourishing experimentation communities or a plethora of think-pieces about how to do it “right.”
We had to hire smart people and trust that they would figure it out.
Most jobs rely on both smarts and experience to succeed. As an industry, we were very short on applicants with experience. So, our prioritization process developed out of trial and error from the smart people here at the beginning of optimization as a field.
Now we can truly say we have a “process” that gets us great results. It’s called the ADVIS’R Prioritization Framework™️ and it works well for teams like ours: outsourced optimization teams who need a lot of transparency, quick results, and a focus on outcomes.
How did you land on this framework?
For the most part, we work with teams who are already running experiments, want to develop a more systematic approach to experimentation, and have oversight from a decision-maker who wants transparency into the process.
So, we needed something that worked well in that context. We developed the ADVIS’R Prioritization Framework™️ accordingly.
What exactly is the ADVIS’R Prioritization Framework™️?
The ADVIS’R Prioritization Framework™ is an acronym for the following:
- Appropriate
- Doable
- Valuable
- Important
- Speedy
- Ready
Some metrics are binary and some use the stoplight system (red-yellow-green).
Walk me through it.
Alright, starting from the top.
Appropriate
Appropriate is a binary metric used to determine if a concept is suited for testing over implementation or consideration.
All of the following filters have to be a “yes” for a concept to go to the next step:
- Is it risky? If there is absolutely no risk, it’s probably not suited for testing and you can assess it for implementation instead of testing.
- Is it in a priority testing area? Many people will have ideas about what needs to be “solved”, so we generally check to ensure that something is within the conversion funnel. If it’s not going to affect conversion metrics, it’s likely not suited for experimentation, because you need something to measure.
- Would it reach significance within the designated time allowance? Every team has a different tolerance for test duration. I know teams that will let a test run for six months, and others that only want to prioritize initiatives that will see significance in two weeks. Having this litmus just assures folks are talking about their tolerance up-front.
Doable
In this step, we ask, “are we capable of running this experiment?”
Sometimes a test is simply not feasible. Maybe it’s not feasible due to platform constraints, or the load time would be too impacted. If the answer is a no, then full stop.
It’s time to pivot to a measurement method other than A/B testing. It might be more suited for rapid testing, so you can still get validation, but it’s not going to be tested on the live site.
Once you determine that an initiative is appropriate for experimentation and doable, then you can move on to assessing the value, importance, and speed.
Valuable
The first stoplight metric in our framework is value.
Value is often the first thing folks think about when they think of experimentation. Most higher-ups think the primary benefit of experimentation is that, if done correctly, you get measurable data to confidently assess the value of a new treatment or mitigate the risk of loss.
Some companies like the Wall Street Journal have a simple calculation they run to determine value. Our value assessment does something similar.
We determine value based on four factors:
- How many users will see the treatment? More is generally better.
- Does the test cater to high-intent users? If the test is very top of the funnel, like on blog pages, it’s never going to be as valuable as a test on product pages. We prioritize tests that occur where purchase decisions are more likely to be made.
- What is the potential lift in KPI? Once you’ve been testing for a while, it becomes easier to determine the potential impact your experiment might have. We use online calculators to weigh potential uplift against audience size, but just knowing that a 1% lift to a large, high-intent audience is more important than a 1% lift in an equally sized, low-intent audience is a good start.
- How much evidence do we have that this test might win? Based on our experience there are certain tests that almost always are a slam dunk and others that are unproven. If we’ve seen something work before, that’s a good reason to give it a higher rating.
Through the value-scoring exercise, we prioritize tests that have a demonstrated track record and will move the needle for the most users in a high-intent stage of the conversion journey.
Importance
While value to the business is, of course, what business is all about, solutions only work if they work for users. So importance is the next stoplight score in our system.
Importance takes into account both how impacted users would be by the change and what we might learn via this initiative.
A test is considered important based on the amount of evidence showing that the current digital experience hinders the UX and the potential to learn about the audience as an outcome of the experiment.
To determine importance we consider:
- Will we learn something new about our audience?
- Will this support decision-making on a near-term initiative?
- How much evidence do we have that this is an issue worth solving?
- Do we have loud detractors?
Measuring these things when we quantify “importance” ensures we don’t lose sight of customer experience in pursuit of revenue.
Another firm might not think of user needs as “important”, but one of our core values is “impact over income”, so we take user satisfaction, ease of use, and accessibility seriously.
Measuring the mounting evidence in support of an initiative is our way of staying connected to the people on the other side of the screen and advocating for people who aren’t in the room.
Speed
The last stoplight score in our framework is speed.
Speed score answers the question, “How long until we see the “value” analyzed in the value analysis step?”
It’s a blended time calculation that takes into account both labor and opportunity costs.
When we are thinking about the speed score we consider:
- Total time investment:
- Asset collection & collaboration
- Design
- Development
- Time-to-significance
We don’t actually assign a numerical value or number of hours to speed because, borrowing from the Agile discipline, we know that people tend to have variable sense of time, and that people get a little skittish when they sense their time is being monitored closely.
So instead, we just use a simple litmus: fast, slow, or average.
We keep it simple because all we want to do is assess the investment being made. Assuming that any amount of time spent on one initiative is borrowed from another, all else being equal, we would weight a fast initiative higher in our roadmap.
It’s also a keen metric for an agency to keep an eye on because, in our experience, clients don’t like waiting around for development to manifest a prototype or for results to come to significance.
Readiness
The last step in our method is to assess readiness. This one is again binary. It’s basically yes/ no based on whether or not we have all the assets and there is roadmap conflict.
After doing this for so many years, we recognized that things were getting held up in development because an antsy team member was pushing them through too early.
Readiness is a good one to keep on the checklist because it helps you slow your roll a bit and not get projects hung up midstream due to missing assets or test audience conflict.
When we assess readiness, we:
- Check for Location & Targeting Conflict — We always stratify the test targeting so that there is no page-level conflict. We would not run multiple tests on the homepage at the same time, to the same audience, for instance.
- Asset readiness — We recommend against prioritizing a test that does not have the appropriate assets ready to be developed. For instance, if a new set of product images will be needed to run the test, we wouldn’t prioritize that test until the images are ready for production.
Wouldn’t it be easier to use a numerical system? In the ADVIS’R Prioritization Framework™️, you’re scoring some items as a binary (yes/no) and some as stoplight (red-yellow-green).
It’s all about optimizing for the desired outcomes and creating an amazing teaching tool.
We’ve found that the rigid numerical scorecard methods are too simplistic and don’t take into account the appetite of our stakeholders. Plus, practitioners hate them because it takes away their autonomy.
Our goals as a consultancy and partner are to make visible progress, get results, and improve the end-user experience.
The best thing about the ADVIS’R Prioritization Framework™️is that it teaches practitioners how to think rather than telling them what to do. The stoplight system spurs conversations about difficulty, feasibility, user needs, and the trade-offs and opportunity costs of any decision.
Obviously, initiatives that are scored highly in all three (value, importance, and speed) easily float to the top.
But it’s what you do next that makes a good strategy, and there’s freedom in working each lever based on your company culture, priorities, organizational maturity, etc.
And how do you use the scores to prioritize?
At the beginning of an engagement, speed is how we earn trust. Showing tests launched and closed tends to quickly prove that we’re working when we say we are, so we generally weigh speed a little more heavily early in a relationship.
But we can’t defer high-importance and high-value initiatives for long because ultimately we’ll be measured both on tangible outcomes like revenue earned and how much better the site “feels” which is a proxy for change. Flashy changes don’t tend to be speedy.
In general, our approach is to stratify tests to cover the bases:
- Have at least one project at a time that is high speed which shows progress
- Work on something high value so we’re progressing toward tangible metrics like revenue won
- Progress on the important things that are flashy and satisfying and that users are most likely to care about
We find that stakeholders are usually the most satisfied when we see a blended mix of high-value, high-importance, and high-speed initiatives in the works.
Why does the ADVIS’R Prioritization Framework™️ work for you?
It helps program managers understand what makes a good test (appropriate and doable), assess opportunity cost (between value, importance, and speed), and only prioritize tests that are truly ready.
Our prioritization method is literally called ADVIS’R, partially as a nod to the fact that it works great in an outsourced context where we need to incentivize visibility, progress, and outcomes.
It’s not the simplest process, but it gets us our desired results, so we love it!
What’s your advice to teams in selecting the best prioritization method for their context?
Do what works. Change it up if it doesn’t. Forget dogma.
The best way to find a good fit prioritization method for your experimentation practice is to just try something. Everyone can keep offering you advice, but unless you actually trial and error the method, you won’t know if it fits your organization’s needs.
If you’re interested in working with a digital experience optimization firm to prioritize and keep you on a clear path forward, we would love to hear from you. Contact us.
Enjoying this article?
Subscribe to our newsletter, Good Question, to get insights like this sent straight to your inbox every week.
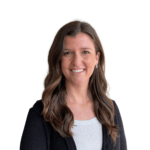
About the Author
Caroline Appert
Caroline Appert is the Director of Marketing at The Good. She has proven success in crafting marketing strategies and executing revenue-boosting campaigns for companies in a diverse set of industries.