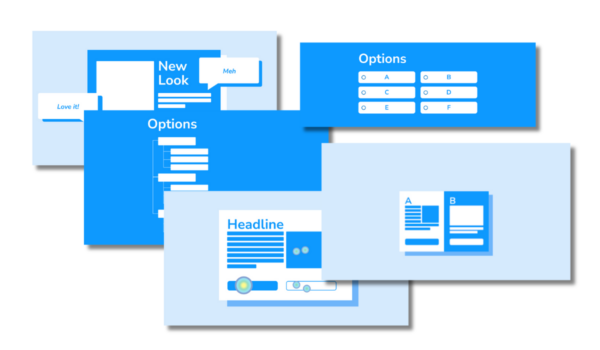
How To Cut Test Cycle Time In Half Without Losing Out On User Insights
Learn how SaaS companies can reduce test cycle time to make the adoption of experiment-led growth practices more accessible.
Experiment-led growth has been quickly absorbed into the SaaS vernacular. The approach complements product-led growth and puts systematic experimentation at the core of decision-making and growth initiatives.
Product leaders have seen firsthand how testing changes with real users before full implementation can minimize risk and maximize ROI. Booking.com, Netflix, and Amazon have made experiment-led growth central to their success by running thousands of experiments annually to optimize UX.
But, teams that don’t have the time, resources, or traffic of the industry leaders might find it difficult to take on the practice effectively.
In this article, we’re sharing how SaaS companies between product-market fit and scale can cut down on test cycle time to make adoption of experiment-led growth practices more accessible.
What is test cycle time?
In optimization, test cycle time is the full length of time taken to complete an experiment. It includes all phases, from ideation to planning, through execution, and analysis. It’s an important metric for measuring the efficiency of testing programs and identifying bottlenecks in the experimentation workflow.
How slow test cycles are holding you back
Slow testing cycles aren’t just annoying, they can create real problems for your business and, as we mentioned, hold you back from the benefits of experiment-led growth.
We’ve seen firsthand with clients how test cycle delays can mean months between identifying an issue and implementing a solution. That’s simply too long in today’s market.
Here are a few of the high costs you might be paying for a slow test cycle:
- Market opportunities slip away while waiting for test results
- Competitors gain ground during your lengthy testing phases
- Development resources get tied up in prolonged testing initiatives
- Customer frustration builds as issues remain unfixed
- Decision fatigue sets in as teams debate what to test next
Significantly reduce test cycle time with these tips
So, what should you do about it? We reached into our tool chest and pulled together the strategies that have worked for our clients and trusted partners to reduce test cycle time.
1. Supplement A/B tests with rapid tests and qualitative research
A/B testing has its place in product optimization, but there are plenty of ways it fails.
Regulatory challenges, low traffic, time constraints, and other issues make A/B testing an at times untenable solution for validating changes to their website or app.
In some companies, by the time a test idea passes through all the bureaucratic loopholes and oversight at an organization, it’s no longer lean enough to justify testing. Without an alternative testing method, teams are left without any data at all. In these instances, A/B testing is just too clunky to make sense.
But, there is a great alternative solution that can provide insights when A/B testing isn’t an option. Using targeted qualitative methods like rapid testing helps overcome the challenges mentioned and builds confidence in changes within days instead of weeks.
Software Director of Product Marketing Gabrielle Nouhra, who leverages The Good for research and experimentation support, says this about rapid testing:
“The speed at which we obtain actionable findings has been impressive. We are receiving rapid results within weeks and taking immediate action based on the findings.”
How it works:
- Recruit users from your target segment
- Choose the right rapid testing method and conduct tests on specific features or flows
- Analyze patterns immediately rather than waiting for statistical significance
- Implement clear findings quickly while planning more extensive testing only where truly needed
In addition to the efficiency of rapid testing and its ability to overcome regulatory challenges, it also has the cool added benefits of adding some qualitative feedback and the voice of the consumer to your work.
For more on rapid testing and how it differs from A/B testing, check out this deep dive.
Enjoying this article?
Subscribe to our newsletter, Good Question, to get insights like this sent straight to your inbox every week.
2. Run parallel tests
Rather than testing one hypothesis at a time, well-thought-out testing programs can run multiple tests simultaneously across different parts of the product experience.
This can be done with both A/B tests and rapid tests.
The most important consideration for running concurrent tests is to differentiate. Our director of UX and Strategy, Natalie Thomas, says:
“It’s important to look at behavior goals to assess why your metrics improved after a series of tests. So if you’re running too many similar tests at once, it will be difficult to pinpoint and assess exactly which test led to the positive result.”
To make sure parallel testing is done correctly, there are a few tips that can help:
- Create a testing roadmap that covers independent areas of your product
- Build small, cross-functional testing teams assigned to each area
- Use a centralized dashboard to track all ongoing tests
- Establish clear handoff processes so development teams can implement findings quickly
When done well, parallel testing can double or triple your insight velocity without requiring additional resources.
3. Prioritize high-impact tests
Not all tests require the same level of rigor. Smart SaaS companies create prioritization systems that allow critical tests to move faster and, at minimum, reduce test cycle time for the most important experiments.
To create a proverbial fast lane for high-impact tests, there are tons of prioritization frameworks you can pull inspiration from (PIE, ICE, etc.). At The Good, we use the ADVS’R framework, incorporating binary evaluations and stoplight scoring. We like that it encourages strategic thinking and accounts for both business value and user experience/needs in the prioritization schema.
While this is our preferred method, there is no “one size fits all” prioritization method. Regardless of the framework you choose, there are a few important steps to making sure you work on the most important hypotheses first.
- Develop clear criteria for what qualifies as “high impact”
- Create simplified approval processes for these tests
- Allocate dedicated resources to fast-track implementation
- Accept slightly higher uncertainty in exchange for speed, where appropriate
Kalah Arsenault, Autodesk’s Marketing Optimization Lead, implemented a custom prioritization calculator that allowed her team to rank test priority on business impact, level of effort, and urgency. The result was 2x the testing volume.
“We were able to double the amount of tests our team took on within one year. So, from this compared to last year, we doubled the volume of testing with a new operating and prioritization model.”
4. Adopt elements of modular testing
Another more technical way to reduce test cycle time is to adopt modular testing.
Traditional design or content tests start from scratch each time. Modular approaches reuse components, dramatically reducing setup time and saving on potential design and development costs.
To get through the testing cycle more efficiently with a modular approach, here are a few tips:
- Create pre-built design templates for common test scenarios (onboarding, checkout, feature adoption)
- If working on qualitative research, standardize recruitment criteria and screening questions for your ICP, and tweak based on the hypothesis and context of each specific test
- Once you get results, leverage analysis frameworks that speed up insight generation
- Implement consistent plug-and-play reporting formats that make decision-making faster
5. Leverage AI as a research assistant
AI can be a great tool to support you in quicker testing analysis and to point you to the research you should be taking a closer look at.
Tools can analyze user session recordings, heatmaps, and customer feedback at scale, identifying patterns that may inspire the A/B or rapid tests you’d like to run to validate changes.
Some practical applications of AI-assisted analysis include:
- Automatically categorizing user feedback into actionable themes
- Identifying anomalies in user behavior that warrant immediate attention
- Generating preliminary insights that researchers can validate quickly
- Transforming qualitative data into quantifiable metrics
Companies using AI-assisted analysis can move from question to answer much faster by quickly identifying common trends in data or research that require further investigation by an expert researcher and/or feedback from real users.
Speeding up your test cycle means better products and faster growth
There is a big caveat to keep in mind with all of this: there are tradeoffs when you prioritize speed.
A faster testing cycle means more room for errors and statistically insignificant results. While precautions can be taken, there are pros and cons to whatever approach you choose.
For some organizations, a slow testing cycle that reaches statistical significance is worth it for a high-risk change. This may mean they’re fine with a custom, one-test-at-a-time approach.
Also, speeding up test cycles takes a certain level of expertise and nuance. You can’t just ask users what they prefer and call it a validated improvement.
To make these changes well, it takes some training, but it’s worth the investment.
SaaS companies that successfully implement these approaches can see benefits like:
- Accelerated product improvement cycles
- Higher customer satisfaction and retention
- More efficient use of development resources
- Competitive advantage through faster innovation
- Improved team morale as they see results from their work more quickly
If you aren’t sure how to get started, let’s talk. We can help you accelerate your product improvement cycle without sacrificing the quality of insights.
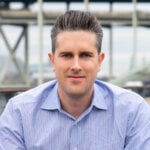
About the Author
Jon MacDonald
Jon MacDonald is founder and President of The Good, a digital experience optimization firm that has achieved results for some of the largest companies including Adobe, Nike, Xerox, Verizon, Intel and more. Jon regularly contributes to publications like Entrepreneur and Inc.